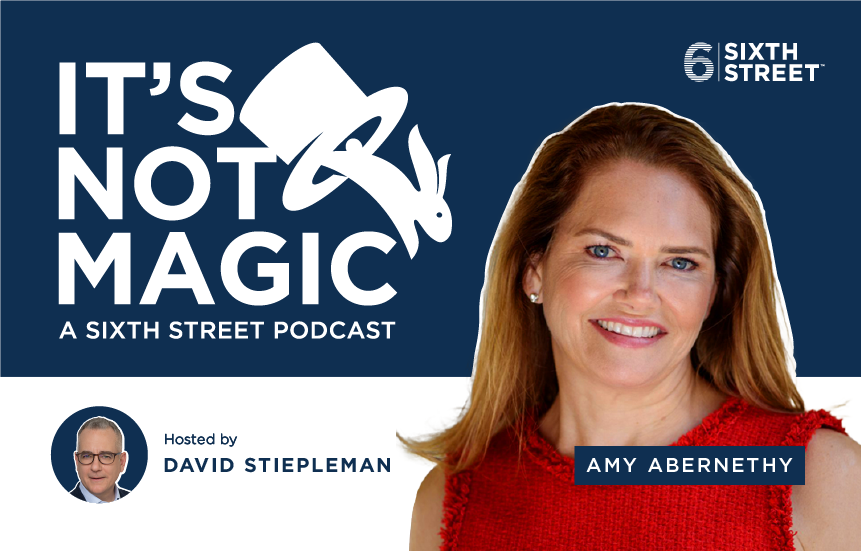
Amy Abernethy, President of Product Development and Chief Medical Officer of Verily
Dr. Amy Abernethy is breaking boundaries in the healthcare space. First by leaving her mark at the FDA and now at Verily, which is part of Alphabet. Join us as she talks about what she hopes to accomplish next by shaking up the traditional face of healthcare.
Amy Abernethy, President of Product Development and Chief Medical Officer of Verily, is an esteemed hematologist/oncologist and palliative medicine physician with more than 500 publications to her name. In addition, she was recently appointed as one of Sixth Street’s Scientific Advisory Board members, working closely with Dr. Jennifer Doudna and other esteemed scientists where they collaborate to help Sixth Street’s healthcare team explore and invest in opportunities related to the cutting-edge gene-editing technology known as CRISPR. Amy previously held roles at Flatiron Health – Chief Medical Officer, Chief Scientific Officer & Senior Vice President for Oncology – before serving a noteworthy stint as Principal Deputy Commissioner & Acting CIO (Chief Information Officer) at the United States Food and Drug Administration (FDA).
No one can deny that Amy Abernethy is making waves throughout the industry by transforming both methodologies and mindsets through her impressive body of work. Our conversation today delves into the effects of the global financial crisis, Amy’s previous experience with FDA regulations, as well as her current focus on collecting real-world clinical data to measure treatment efficacy. We also explore where she envisions Verily headed in patient care and how it will continue to shape her career path.
We are glad to have Marty Chavez, Vice Chairman and Partner of Sixth Street, join us for our episode. Marty is a member of the Alphabet’s Board of Directors and Scientific Advisory Board of Sixth Street. He is also the chairperson of various boards such as Broad Institute MIT & Harvard, Stanford Medicine Fellowship Program, Recursion Pharmaceuticals Inc., Cambrian Biopharma, Earli, and amfAR (the foundation for AIDS Research).
Listen to full episode:
Listen On:
More from this episode:
- Reflecting on the Promise of Real-World Data, a Fireside Chat with the FDA, Syapse, November, 2020
- Evidence Accelerator Model – A Critical Catalyst Towards A Learning Health System, Reagan-Udall Foundation for the FDA, April 23, 2021
- Here’s why Abernethy wanted to jump on board at Verily and her ambitions to grow its research business, Fierce Healthcare, June 14, 2021
- Verily’s new big hire, Amy Abernethy, on her plans to transform clinical trials — and bring in money, STAT, June 3, 2021
- Regan Udall Foundation for the Food and Drug Administration
- Acela Amtrak
Episode Transcript:
David Stiepleman: Hello. Welcome to ‘It's Not Magic’, a podcast from Sixth Street about business building that strips away the pretense and gets right to the useful stuff. I'm your host, David Stiepleman. We use this show to talk to founders and industry leaders to get them to explain in plain English what they set out to do and specifically how they do it. We're excited for every episode, and that's especially true for this one.
Amy Abernethy: The chance that those new treatments that we were developing, make their way into the clinic in a timeframe that the person sitting in front of me could benefit from was really overwhelming. That just was never going to happen and most of the people that I was taking care of were like 30- 40 years old. And so I started to try and figure out what can we do to accelerate that process?
David Stiepleman: That's Dr. Amy Abernethy, who from 2019 to 2021 was the Principal Deputy Commissioner of the Food and Drug Administration here in the United States. That's the top non-politically appointed seat at the FDA. She's now President of Product Development and Chief Medical Officer of Verily, a health technology company that spun out [of] Alphabet. Amy has also just joined Sixth Street’s Scientific Advisory Board. She's an oncologist by training, has run an academic hospital, worked in venture, steered the FDA through the pandemic, and is credited with modernizing the FDA's approach to data. We'll talk about all this and much more with Amy. I say we because the extra bonus here is that I was joined by Marty Chavez, my partner at Sixth Street and full disclosure, he's also on the board of Alphabet. He was a successful Wall Street executive who of course got his PhD in medical Information sciences and is known for turning the traditional way trading businesses got done into software businesses.
David Stiepleman: He's going to describe what that actually means. Marty works closely with our guest, Amy, with Dr. Jennifer Doudna and the other scientists on our scientific advisory board as they help Sixth Street’s healthcare team explore and invest in opportunities including CRISPR – little callback. You may remember my conversation about CRISPR with Janice Chen of Mammoth Biosciences back in season one with Amy. You'll hear as we explore the parallels of using real-world data to model and solve complex problems in life sciences and finance, how you learn to spot patterns and how you solve problems and a lot more. Okay, buckle up. This is a super fun conversation and an important one about science and healthcare in life, but it's not magic.
David Stiepleman: Dr. Amy Abernethy and Marty Chavez. Marty, you're a doctor too, but I've known you too long to do honorifics. We can skip it. Thank you so much for being here. I have an opening hypothesis, which is a little bit prewired with Marty. I think that the two of you, Marty, in running Goldman Sachs and spending your career automating software of Wall Street and Amy doing similar things in life sciences and healthcare. I think you've had similar paths. That's a hypothesis. So, in getting at that hypothesis, I'm going to start with you Amy, if I could, what are real-world data? What does that mean?
Amy Abernethy: What are real-world data? Well, ultimately when I think of real-world data, it's data and information that reflects how people function and treatments work in the settings within which we live. Sometimes these are data that are already being collected for another purpose, such as electronic health record data or administrative claims data. Other times these are data that we're intentionally collecting prospectively, such as pragmatic clinical trials or patient reported outcomes. And then there's this emerging set of real-world data that I think is also really important, such as molecular data from tumor samples that can act as key information or real-world data set or sensors in a watch that can help us understand mobility or social determinants of health. So all of these help us put healthcare interventions into perspective for the real world.
David Stiepleman: Tell me why that's important. Why have you been focused on this? We're going to talk about your career, which is incredibly varied in some ways, but is incredibly consistent in other ways – you've been focused on this forever. So why is that important?
Amy Abernethy: Well, when we think about a clinical trial, traditionally what we do is set up a set of experimental conditions and experiment almost like a terrarium, right? That simulates the health of individual people and how a treatment might impact their health. But we really do set up completely experimental and non-real-world conditions, yet when we think about prescribing a treatment, we want it to work for real people who need to go to work and who have to take care of their children and may have another comorbidity or another condition and worry about our side effects going to change my life. And so really as we think about what we want to know about a treatment, it's not the terrarium story, it's actually the story in the context of natural life. And so that's always been my motivation. There's also something else that's motivated me, and we'll come back to this I suspect, which is that can we find ways to accelerate the process of understanding what works for whom and when? And one way of doing that is actually leveraging all available data instead of recreating every data point anew for that experimental terrarium. And so the other advantage of real-world data is it acts as an accelerant, a short circuit, so to speak, of conducting evidence generation.
David Stiepleman: Marty. Sound familiar?
Marty Chavez: It sounds familiar. To draw a parallel, Amy, and this is one of the ways in which we connected so powerfully when we first started speaking, is we had the same problem or parallel problem on Wall Street. And the way we approached it is, of course, we're going to be doing trades with our clients in the real world. At the same time, we're going to be building a parallel software world or a digital world where we can then do all kinds of experiments that would be dangerous or impossible in the real world. We can ask all kinds of counterfactual ‘what if’ questions and lose a lot of money in those simulated worlds so that you can do something about it in the real world and avoid losing money in the real world. So, there's this interplay between the terrarium and the real world.
Marty Chavez: And you need them both, I think is where we came out, right? If you're just blithely operating in the real world without conducting any of these software or imaginary experiments, that's going to be problematic because you're going to miss things you're going to fail to see around corners and that's what's going to come out and bite you. At the same time, when we saw this with some of our peers on Wall Street, if you're believing your model too much and you're believing in those parametric distributions, that's when you're really going to get killed because the real world is not like that imaginary world.
David Stiepleman: I feel like you both have had to have dealt with. I know you've dealt with the integrity of data issue. So, Amy, maybe can you talk about that, very important to know what you're looking at and very important for a regulator when you were at the FDA to know what the FDA's looking at. How have you started to tackle that problem or how have you been tackling that problem?
Amy Abernethy: Well, to start with, as I was listening to Marty, I was reflecting on the fact that this interesting crossover between the experimental world and the real world is often through data, which therefore requires technology and also provides the opportunity to create sophisticated analyses or leverage artificial intelligence on top of the data. And so, data becomes this bridge, and as you highlighted, data integrity then becomes a really important feature because practically speaking, if data is the bridge and we're going to rely on it to do a lot of sophisticated things, then the questions around whether or not the data themselves are reliable is a critical issue. And now think about how messy healthcare is. Healthcare is messy, not just because each one of us is our own unique flower, but also because there's lots of reasons that errors get introduced into the healthcare system.
Amy Abernethy: Some of them are just cut and paste errors and transcription errors and the fact that I'm moving really fast when I'm in the clinic. Other ones are the fact that two doctors looking at the same patient often see two different things, maybe even two different diseases. And so even categorization of disease is hard. And then you layer on yet another set of issues, which is that some findings are very objective, like measurements, for example, about a genome. And other findings are very subjective, such as a person's report of pain, nausea and fatigue. And so, all of this gets mixed up together, made worse by the fact that if you have ultimately data coming from many different places, they’re often in conflict and we don't have an easy way of knowing which ones right. So, regulators get worried because how do they audit or confirm findings? And people who have historically worked in that terrarium world get worried because they're used to creating really, really careful stories, experiments that get the data as high quality as possible. And so, practically speaking, if we're going to leverage data in new ways to create that bridge, then figuring out capabilities that are needed to be able to have confidence in the data and ensure that they're reliable, is going to be the task of the future.
David Stiepleman: You and your colleagues at Verily, I think recently wrote a paper on another problem or possible problem with data collection and data integrity, and that's racial bias. And you hear about this when you talk about AI more generally, like, ‘hey, if we're just building bias into these systems, that's going to be a huge issue for our society.’ Talk about that paper or talk about how you're thinking about approaching that.
Amy Abernethy: Yeah, so you just highlighted one pretty critical issue, which is that, again, if we're going to leverage data to be able to create that bridge and then the data are unreliable or biased, then that bias gets baked into our algorithms and then baked into the decisions we make in our decisions actually then amplify bias in the system and we certainly see that with respect to race and diversity and a person's background. Another aspect is that the data themselves can be biased because we under-represent certain people, or we allocate or categorize people without really thinking about the full distribution of who a person really is. And then also the way that we deliver healthcare, the way that we conduct clinical trials that terrarium et cetera, makes it hard for people of certain backgrounds to even participate.
Amy Abernethy: Whether that is because participating in clinical trials requires you to get on a plane or drive a long way to participate, and that may leave certain people out of the process, or that participating in a clinical trial means that you have to take days off from work and certain people can't do that or healthcare delivery happens differently in a rural health center than it does at MD Anderson. And so, all of these things amplify in the system. And so it apparently, we've really made a kind of transversely commitment to health equity building software and capabilities that are easy to use and low burden for all people, and then looking for bias in our data sets in order to reduce the bias in our algorithms.
David Stiepleman: By the way, maybe we can represent the hypochondriac community of which I'm a member, so we can talk about that at some point, , but that's a little bit from what you're talking. Maybe we don't want to waste time on this . I do want to take half a step back or actually a couple steps back. And you alluded to this, your career, once you were a doctor, you're an oncologist, a clinician, an academic, you were in venture, you went to the government, now at very well-funded, but still kind of startup-y sort of thing. What was the moment, and I wonder if it was when you were with patients, what was the moment where you were like, ‘we got a problem here, we got to fix this, we got to figure out how to get reliable data more quickly through the system so we can get cures into people more quickly and more reliably.’ Is that right? And what was that moment?
Amy Abernethy: Yeah, so I'm an oncologist by background, as you said, a melanoma doctor. And I used to be in the clinic, and I'd be sitting in the chair in the clinic talking to the person in front of me, oftentimes talking about a chance of living five years of being something less than 50%. And yet we were working on diagnostics and vaccines from melanoma and new treatments in the labs all around us. And the chance that those new treatments that we were developing would ever make their way into the clinic in a timeframe that the person sitting in front of me could benefit from was really overwhelming. That just was never going to happen. And most of the people that I was taking care of were like 30-40 years old. And so I started to try and figure out what can we do to accelerate that process?
Amy Abernethy: We can't just leapfrog and bring treatments that don't work or are unsafe or are unjustified into the clinic. So, we've got to actually make sure that we are conducting evidence generation in thoughtful ways. And so that was important that we actually had to accelerate evidence generation, but we also had to figure out like how do we accelerate discoveries themselves and what could we do on that side? And then the other piece of this, which is access. If we developed treatments that work, but nobody can pay for them, or you actually can't get them to the people who need them, that's also part of the problem. And so I really started working on how do we speed up that process and really worked on it from every angle finance and sort of thinking about how do you make sure that there's adequate capital science data and technology regulations. And so pretty much if you look at my career, it's really trying to push on this from every side to try and help solve that problem.
Marty Chavez: Amy, as I'm listening to you, I have to jump in and ask what are some of the advantages and disadvantages that we have specifically in the United States in our healthcare system. And when I was a med student at Stanford a million years ago, we were working on the electronic medical record. We're still working on it. Other countries such as the U.K., at least ostensibly, have a much more unified system of data. But we've got all these providers and all these payers and every time I go to a new specialist, they give me a clipboard with 30 pages to fill out and ask me to write my vaccine history going back to my birth , which I have absolutely no clue on. So, what are some of the problems specifically in the U.S. or some of the advantages that we have?
Amy Abernethy: So let’s start with the advantages. There is an advantage to our entrepreneurial spirit, our ability to test things and say, ‘that doesn't work’ and then move on. There's also an advantage to our scientific investment and our ability to bring that investment, especially in the basic sciences, into the clinic. And our ability to leverage capital, none of which by the way is like healthcare delivery in itself. And so I think when we come to the pointy edge of healthcare, so the management of cancer and rare diseases, just new discoveries that are for people who are suffering from many of those things, the ICU, we really do see lots of American innovation show up across that space. Then you go to what you were just pointing to Marty, which is the disadvantages.
Amy Abernethy: And I did my PhD in Australia, so I witnessed healthcare from an Australian perspective, which is mostly funded with a small top up from an insurance point of view and much more universal healthcare. And what I could see is that first of all, access, which is making sure that at least the basics get to everyone, is something that we miss here in the U.S and many times, it goes back to that issue of bias. So, then that actually amplifies bias in our system because there's challenges with access. But as we think about this issue of data, places like the U.K. can leverage their investments in the national health system, not only for healthcare delivery, but now leveraging those data to start to accelerate evidence generation.
Amy Abernethy: And we saw this in the context of Covid in two ways. So, the NHS now in the U.K. is the country where the recovery trial happened, which was essentially a single platform trial evaluating multiple treatments simultaneously against Covid, and then rolling off the printing press, so to speak, our knowledge about one after the other. And then similarly, there's something called Health Data Research U.K. or H.D.R.U.K that really brings together all of the U.K.-based data sets to try and stimulate discovery. And that got brought into action in the context of Covid. And so, you can see that that national investment and national connectedness shows up in how it can help and the pandemic brought that to light.
David Stiepleman: I have maybe a meta question for both of you. So, Amy, you're describing your career and you're pushing on this problem from every possible angle in clinical, and then you're getting research out there, making sure the data is evaluated by regulators in every possible angle. Most people don't do that, right? Most people are, you know, they're a doctor, they're going to figure out how to handle their patients or maybe they become a researcher but you're going into every possible organization you can to try and figure this out. What is that? Is it a habit of mind that you keep asking that question, ‘wait, okay, I want to go solve the next problem, the next problem.’ What are you doing over the course of your career that you just are just, ‘okay, I'm going to go relentlessly push on that, I'm going to go relentlessly push on that.’ But you're seeing the field. It's very impressive. How are you doing that?
Amy Abernethy: Thank you. And I guess it is kind of a meta question. So, you know, as you were asking it, I was thinking, one side, it's really relentless work to try and push a real problem that's in front of me forward and make progress against it. It's required a couple of things. One is when there's the suggestion, you can't do that because you're not trained for it appropriately or you can't make that mid-career change – just sort of ignoring that and figuring out how do you keep going forward? I think there's a second piece which is – practically speaking – in any one career, right? There's a path towards the pinnacle, whether that's winning the Nobel Prize in one direction and having a spectacular exit in another direction and sometimes you have to be willing to forego that path to the pinnacle in order to make a horizontal shift in order to keep pressing on things from a different direction.
Amy Abernethy: But I would say that something else you said really caught my attention, which is that it is aggregate or synergistic that the more that you shift and push on the problem from yet another direction you bring with that shift what you've learned in the past. And now I can become, a person at the FDA, literally leading the FDA going, ‘wait a second, I know what tech companies look like and the challenges that they're going to have and can think about incumbents versus startups, et cetera.’ And think about now how do I push that forward because I've actually seen that now showing up at the FDA and now that I'm at Verily I can sort of think of all I know what regulators worry about and how we do something in a way that relieves some of that angst so we can actually move the ball forward.
David Stiepleman: Do you get increasingly comfortable as you make these shifts? Like, ‘okay, I'm not supposed to know what I'm doing here, but I've done this before ,’ ‘I've done the I don't know what I'm doing before.’ And you get better at it.
Amy Abernethy: Yeah. You know, part of it is you start to realize, I mean, some of this a little bit of imposter syndrome all along the way, right? You start to realize everywhere you go, people are not entirely sure of exactly what they're supposed to be doing or whether or not they're really supposed to be there. And you get more and more comfortable sitting in a room, listening, watching people's body language and how people navigate and use those inputs. Probably more than textbook and degree inputs.
David Stiepleman: , we might have video in season three, but people can't see you. Marty, you're shaking your head with some degree of recognition. . What are you thinking?
Marty Chavez: Well, I'm just thinking, as I listened to Amy and this is one of the ways in which we first connected, I have also jumped around a lot. Sometimes people look at what I've been up to, they say, ‘wow, you've done a lot of different things.’ And they're actually probably thinking, ‘wow, it sounds really confusing and random’ . But it isn't like I was so bold and jumping into one thing or some different thing because to me, I've always really been doing the same thing just in some different problem domains. And in reality, I only have a couple of tricks and David and I have been working together for a while, so he's already seen them all. But one of them is ask a lot of questions, gather a lot of data, keep all the data in one place, and then start building models on the data and then use the models to generate some more data and just keep bootstrapping with the data to ask more interesting questions and get some more answers.
Marty Chavez: And then never throw anything away. Keep it all in one place so that whenever you're wondering, ‘where did I put it,’ you know that the answer is ‘I put it in the place where I put all the data and all the models and you just kind of keep doing that.’ And I found that it's a trick, if you will, that works again and again. It worked in the early days of the trading business at Goldman Sachs. It worked in the depths of the financial crisis when we could turn to all those models and data sources and we could see across the business and across the clients in a way that few people could. And now in the world of alternative assets, we're doing the same thing. We're looking for companies that are also doing that same kind of thing in their business, gathering data, building models, deriving more data and bootstrapping that and so it really is all the same thing.
David Stiepleman: I want to talk about crisis and I don't want to equate the GFC with Covid, but maybe to piggyback off of something, Amy, you said you went to the regulator, you realized, ‘hey, this is what VC firms are looking for and how can we be helpful and how can we leverage the things that I want to leverage to make the regulator, -- by the way, the FDA, which regulates so many things and as a pace setter for the world, -- you should talk about that. But you were in the seat, I think for – if I'm right – about a year when Covid hits and the convening of these collaborative settings with data scientists, with health experts, also your home, presumably. How did you do that?
Amy Abernethy: You know, if I look back, and I'm going to tell you a very funny story just because it sets the stage great. We have now all gone virtual and I kept saying ‘I have to have a whiteboard because I can't figure out what to do.’ And I've been walking and talking on the phone, trying to find people to help identify data, et cetera and my husband takes white plastic and puts it over some kind of ironing board thing and sets it up on my back porch and says, ‘okay, you have a whiteboard now solve the country's problem.’
Amy Abernethy: I still have it, and what the point that we were trying to figure out is how do we bring people who have data together with people who can analyze data together with people who now will be able to take those results and use them quickly in a way that short circuits the cycle because if it's going to take a year or three, that's not going to cut it. And this was March-April of 2020. And so, what we did was we brought together health tech companies, academics, we brought together government and researchers of many different types and built what we called the evidence accelerator. If we wanted FDA to use the information, they couldn't convene those organizations. So, we leveraged a congressional mechanism.
Amy Abernethy: And this goes back to that point. The more you start to learn where are the points that you can move things forward, and we found this congressional mechanism we could leverage call the Reagan-Udall Foundation and then got people meeting every week and showing each other, ‘here's what my data can do, here's the analyses I need to run.’ And then having regulators say, ‘here's what I trust, and I don't trust’ in real time. And every week they would show up with the next set of results. The reason that that was in my mind so powerful is first of all, it accelerated the regulator's understanding of what real world data could and could not do. It accelerated tech companies and academics and health systems and researchers’ understanding of who held what capability and where they could partner. Some of those partnerships are going on now and have led to new companies and new analyses. And it under accelerated our understanding of rural data overall to inform now, for example, the guidance that the FDA wrote at the end of 2021 for guidance that actually outline how FDA thinks about rural data and how they think about potentially using this for regulatory decisions into the future. And what it really required was the forcing function of getting everybody together in one crucible and moving that quickly.
David Stiepleman: One of the things that we focus on at Sixth Street is – we call it cross collaboration at scale. The scale that you're we're dealing with within the FDA, presumably across agencies, across countries, was there a method that that worked better than other methods in terms of how did you start a meeting? How did you invite people to meetings? Were there sort of tricks where you could make it more likely that there would be transparent collaborative spaces?
Amy Abernethy: So, I was reflecting when Marty was talking a few minutes ago about collecting data, making sure everything's in one space, and leveraging things that he'd learned in the past, the beginning parts. And the reason why I described that whiteboard, the beginning parts were getting on the phone with as many people as I could think of. And every single time I would be on one call I would say, ‘and who else do you think I should talk to?’ I'd write on that whiteboard on my back porch, three more names. And then I'd say, ‘well, can you give me their phone number? And then I’d get on the phone with those folks and basically said, ‘here's what we're thinking about as FDA, here's what we think is, you know, going to really be important in the government. What would you be willing to bring to the table?
Amy Abernethy: And then started actually calling people to say, ‘okay, I want you to present Thursday night at 5:00 PM please be there. I'm the FDA.’ And really pushing those conversations forward so that people heard what they had and what they were thinking was important so that we showcased one group to the other group. So, then all of a sudden that showcase it casing created both the sense of important and a bit of FOMO, and then also really being explicit about ‘here's what we've learned and here's how we're going to take that forward in a way that people then felt like what I just did had value.’ And by continuously doing that, what was interesting is it started to spread not just in FDA, but we then had the same conversation happening in CDC, and then we were having these conversations with NIH, and then we were talking to the NHS in the U.K. and then we were talking to the EMA in Europe. And what was really powerful in my mind was that people could see if I show up, I can create and contribute, in a way that actually is meaningful.
David Stiepleman: Marty, any thoughts? Do you remember back in the crisis when everyone thought the world was going to explode and you were trying to talk sense and understand risk and getting people to talk to each other and not hyperventilate?
Marty Chavez: Well, , you're really taking me back and is I remember traumatic experience and actually oddly David, there were some parallels in between the great financial crisis and Covid. Specifically for the financial system. And one of the areas that we worked on tightly with our peers and with the regulators was how can we all put together what we're seeing in our fragmented systems, all of which have a partial view of what's going on and how can we help the regulator piece together everything that is going on, and what are some of the practices that worked really well and some of the things that worked really terribly that we can avoid. And putting all of those together systemically and out of all of that came regulation. And as you both know, I'm a huge believer in regulation and the right regulation.
Marty Chavez: There's a personal view. There's a lot of financial regulation that doesn't serve much purpose. And then there's some regulation that is absolutely essential. And I think out of everything that came from the GFC, there's one piece of regulation that made all the difference and is the reason that you heard about disruptions in absolutely every sector during the pandemic except for the banks. I think that is really remarkable, right? That the banks are usually the first to show signs of massive stress. But really what came out of the GFC was this important notion that Amy, I know you're passionate about, is of data and modeling and simulation. The core regulation is ‘let's have all the banks simulate themselves for nine quarters in the future’, and that's a lot of math and software and a lot of people and a lot of questioning. And then let's give them a macroeconomic scenario that the regulator creates and see how those simulations perform. And based on those simulations, we're going to decide how much capital and liquidity they actually need to hold. And that, to my mind, is the most powerful piece of systemic collaboration with the regulator leading to model building and data collection that actually fed back to make the world a better place.
David Stiepleman: That's fascinating. And, and the theme of that, for me at least, or one of the themes is having real kind of honest brokers in industry, and who are actually talking to the regulator without their handout or without talking their own book and, just trying to get it right. And, if you can create that environment as a regulator, as an industry participant, boy, it leads to much better outcomes it would seem.
Marty Chavez: There I have to ask you, Amy, since you were, you were in the seed, I was never actually a regulator, but I remember after the financial crisis going to DC a lot and was on the ACCELA every week and building credibility with regulators from a place where frankly, we had no credibility with regulators. But we did know how the financial system worked, or at least how parts of it worked. You must have developed a keen eye for people who are going to help you do your job versus people who are narrow…
Amy Abernethy: The first thing I learned was the importance of public-private partnerships. The ability to have well organized, well understood and well moderated safe spaces where government and private industry could come together and exchange ideas. And that was the mechanism we leveraged for the evidence accelerator, which was this congressionally mandated mechanism called the Reagan-Udall Foundation. But what's interesting is there are a lot of people skeptical of those mechanisms. So, because they think about it as the opportunity to essentially lobby government to do things around traditional systems. And the thing that I found so important about public-private partnerships is that it's the opportunity for the regulator to get their head around, to get a look into the possible – where things might be going so that now you can imagine ‘what might I need to regulate in the future, or what tools might be available to me and what am I going to need to know more about?’
Amy Abernethy: ‘How am I going to poke at what I trust and what I don't trust?’ And if it weren't for the fact that we could create those public private partnerships, then there's no opportunity for regulators to see that. Let me give you a really tactical example: we're moving into this space in the development of privacy sparing capabilities such as synthetic data sets to be able to be analyzed and give a reliable output that is so consistent with what would've happened leveraging the real dataset that we could think about using those synthetic datasets now for a variety of tasks, including evidence generation around medical products like drugs. Can you imagine being a regulator and saying, ‘you're going to give me synthetic data and a model…
Marty Chavez: And output?
Amy Abernethy: , you're going to make it up, what am I going to have to understand and believe to be able to trust this to make a high-risk decision like approving a drug in the future? And if it weren't for the private public private partnership space, that space of Reagan-Udall Foundation, there wouldn't be the opportunity for the regulator to even see what's happening on the tech industry side, let alone say, ‘I don't trust that part. Here's what you're going to have to do in order to start to verify that for me.’
David Stiepleman: How have you tried to build into what the FDA's looking at and what data is being generated to avoid this dystopian future where real-world data includes the fact that I self-identified as a hypochondriac and now I can't get a job anywhere, . How do we avoid that because everything's going to get a lot smarter and it's going to be a lot easier to figure stuff out about people and presumably look into their records. What are some of the breaks that you've tried to build into what you're doing?
Amy Abernethy: Yeah, I think this issue of privacy is going to become more and more front and center for a ton of reasons, not the least of which is as we bring in more and more data points, the more data points, the more individual the actual designation becomes, and it becomes that much harder to essentially keep somebody anonymous. I see three critical areas. The first is that we do need to have privacy sparing innovation, and that's why I mentioned even just for example, a synthetic data sets on one side, you know, if you're the regulator, ‘I'm not going to trust that’ on the other side. We need to be able to develop these innovations and figure out how do we trust them. So, we need to work our way through both what are the capabilities as well as how do we need to verify them.
Amy Abernethy: The second is we really need a public conversation that is progressively more sophisticated than where we are today. We have really learned how to think about privacy and the use of information and participating in all parts of our lives and in healthcare, we've been black or white around it. We have said, ‘things must be kept private and there is a no-go zone.’ But then that means that I cannot participate in a number of ways. And we really need to have that conversation in a public way to get as sophisticated as possible. And then the third is that we actually need to also get smarter about how we work within the systems that we already have such as HIPAA or GDPR. Right now at Verily, I spend a lot of time thinking about how do we build capabilities in line with current policy that allow people to have as much transparency as possible and understand what's happening with health data and understand what participating means so that then they can say, I can participate confidently and I also have the ability to pull back my participation if you don't use my information the way that I've previously given you permission to, and that we have the ability to do within our own currently available policy system.
Amy Abernethy: So, I think we need to think about all three of those.
Marty Chavez: Amy, I'm curious, there's some very much on the edge, computer science, secure, multi-party computation, federated learning, all of these privacy preserving techniques, right? The notion that you might be able to train up a machine learning model on a bunch of data without the machine learning model knowing anything about the individual items in the data. Do you see possibilities there, or do you think that's still science fiction?
Amy Abernethy: I totally see possibilities there. And, I've seen a number of directions that I find compelling. The question becomes, I keep coming back to what will we need to be able to have available to us to have confidence in the output in order to make high risk decisions? And I see that in a couple of directions. One is being able to have confidence in how we got to the development of this privacy sparing capability in the first place. The second one is how do we audit it and how do we essentially unscramble everything and crosscheck, a core task. A third is if we're going to rely on novel solutions like privacy sparing capabilities, how do we do so in a way that's not one big decision? And then the whole world has to rest on that decision from that point forward, but rather we make a decision and then we follow the impact of that decision and continuously crosscheck and update across time. And so I do think there is the possibility of starting to leverage these capabilities in the future, but I think we're going to have to both figure out how do we have confidence and, also figure out how do we not let them go off the rails.
David Stiepleman: As you were heading out from the FDA, I think one of the things you said is, ‘look, the things that we've been putting in place, they're starting to work, I can see it, so you don't need me anymore, kind of thing.’ Are the outputs of those processes and that thought leadership, you were engaging in there as a consumer. Am I starting to see that? Is that visible to the amateur like me?
Amy Abernethy: No, you're not an amateur but . So I can put it this way. I remember I was saying something to my mother last night, and so even like my mom has the opportunity to see some of these things. So, we are starting to see more and more activity in the leveraging of real-world data. And I take great, and I'm not going to call it pride, but a sense of ‘thank goodness,’ around that. And as I said, that showed up in four draft guidance’s at the end of 2021, but it also showed up in how we, for example, leveraged the Israeli data to understand the continued performance of Covid mRNA vaccines, including duration of effectiveness. Do you need a booster side effects? So that's one thing that I feel really heartened by is that we are learning how to use data in new ways.
Amy Abernethy: And another is that I was pretty vocal and continue to be that we still need forward-looking clinical research. It doesn't mean that everything has to be the terrarium, right? But rather we need prospective pragmatic research. And, I feel good that we're now seeing that show up more and more, whether that's the oncology center of excellence at the FDA’s big push in, I think they call it program pragmatic or something like that, around leveraging pragmatic clinical trials in the oncology setting. Or it's companies now who are thinking about leveraging pragmatic clinical trials to study their new drugs. I really feel that it is something that has continued. And then the other thing that I worked on when I was at FDA that I can discuss with my mom, and feel good about, is that healthcare is about people and the person, whether that's patient reported outcomes and patient experience and voice, or more patient-centric drug development, or just being able to talk on a daily basis about how important being patient-centric and low burden is to the overall system.
Amy Abernethy: I feel like that is something that has continued since I was at FDA and goes back to that time in my melanoma clinic when that was what it was really all about was how do we take better care of this person sitting in front of me?
David Stiepleman: That's amazing. I'm glad you brought up your mom because didn't you help her write a textbook when you were like nine years old or something? Like – she's not exactly an amateur either, right?
Amy Abernethy: . Oh, wow that's wild you know that. So, when I was a kid, my mom had this idea that you could help nurses come back into the workforce by teaching them dosage calculations, how much you put together to make a dose of a medicine that you give to a patient. You could help them understand that by kitchen math, you know, cup of sugar and two sticks of butter and you dissolve hot cocoa in by putting the powder into hot water. And so my mom wrote a textbook, that still is one of the best, most used nursing textbooks in America. And in fact, the last two editions I've co-written with her. And I learned it at the kitchen table. .
David Stiepleman: That's awesome. The medicine sounds delicious in your house. , yes. . We're doing our research on you and one of the things that came up was a paper you wrote, academic papers – I didn't try. But you wrote something with somebody from Russell Reynolds, the executive search firm, and I thought, ‘I wonder how interesting this will be.’ It was awesome. It's about archetypes of CMOs and I thought, ‘gee, that title, it was super interesting.’ And, it was like this very rigorous way of bucketing different types of people. And what I want to ask you about is hiring and helping people onboard. And one of the examples you had is like, ‘gee, if you're going to hire an academic CMO, be careful. Here's, here's what you got to do’. What works in your life when you're hiring people – getting people on the same page in particular.
Amy Abernethy: Yeah. So, um, , it's fun that you saw that paper. It came about because I was always getting calls, ‘I'd like to hire chief medical officer from my organization and, can you recommend somebody to me?’ And I started asking people about, ‘well, tell me what you want this person to do.’ And as I think about this issue of how do we onboard people, how do we build alignment? One of the most important things is being really clear about what you want this individual to do in your organization as the chief medical officer. So if you want this person to be involved in sales and essentially be responsible for sales enablement as medical leadership, then onboarding them in in a way that helps them align with what commercialization and sales is all about.
Amy Abernethy: But, you know, practically speaking as the CEO, you can't just assume that the new chief medical officer that you're hiring understands all of the elements of what you want your company to look like or what business looks like when they're coming into a new business environment, especially if they're coming from clinical practice or from academia. So really as the CEO work together with your chief medical officer to help them understand the features of what the kind of CMO you're looking for, and then perhaps find the mentors and the environment that also meet that archetype.
David Stiepleman: It's really interesting. It is really giving me a lot to think about in terms of hiring – where are people coming from and what you were just talking about.
Marty Chavez: I think it's great. I just had one thought. So Amy, when I hear about pragmatic clinical trials, I'm wondering what are they the unpragmatic kinds or the idealistic kinds? What's the distinction there and why is it important and useful?
Amy Abernethy: Great question. You know, as I think about pragmatic clinical trials, they're really on a continuum. But from the traditional clinical trial that terrarium we were talking about before does something that looks as consistent with real life as possible. And the kinds of ways that studies become more pragmatic are by widening eligibility criteria. So it makes it almost easy for anybody to get in, perhaps. The only requirement is you have hypertension, and that it's moderately severe. Another way that trials become more pragmatic is that rather than trying to measure every single detail, you stick to just the most important elements to measure such as this tumor growing or shrinking. But then everything else you leave alone in the measurement process. Another way that trials become more pragmatic is that you allow people to do all the other elements of their regular life beyond what the clinical trial is.
Amy Abernethy: So perhaps you're just studying a drug about diabetes, but the person still needs to take their medicines for depression and medicines for hypertension. You allow those other elements to sort of be as per real life. These are all different ways that we go from the terrarium to something that looks much more real life. And the only thing I would say is you can kind of imagine as you allow sort of more variability, the ability to measure precisely starts to reduce. So you reduce that precision and so those are the kind of trade-offs you're making when you develop pragmatic elements.
Marty Chavez: So it's a trade-off as opposed to just the dichotomy of a triple blinded perfect RCT versus everything else. Got it.
Amy Abernethy: Yes. Most pragmatic trials though, are randomized, although it's not a requirement.
David Stiepleman: Last question. Next 10 years, what are you excited about seeing? What do you think is going to happen? Make some predictions or going to science fiction or whatever you want. What are you excited about?
Marty Chavez: Maybe something contrarian and really weird.
Amy Abernethy: , I was hoping you were going to be the first Marty to say what you're really excited about . So the thing that I'm really excited about is that I truly do believe we're going to get smarter about leveraging all available data. And I think that one of the key elements that we're going to develop are algorithms that allow us to put many different data sets together and essentially solve for those underlying data inconsistencies that make the real-world data so unreliable. And that those algorithms themselves are going to be very, very important and very powerful in this whole process going forward. The only other thing I'm going to predict is I have no way to predict what my next career will be. There you go.
David Stiepleman: . That's awesome.
Marty Chavez: I love that self-preferential medical prediction.
David Stiepleman: Exactly. That was awesome.
Marty Chavez: I'm super excited by the breakthroughs of AlphaFold, another Alphabet company and it's really an amazing problem. I was one of those hundreds of thousands of poor students who did those protein crystallization experiments, in my case, not very well, but produced the training set that ultimately enabled AlphaFold to predict 200 million amino acid sequences in how they fold. And that's amazing. But if you think about the bigger prediction problem, we have a patient with symptoms, manifestations, findings, however the patient presents, what's the drug that's going to make the patient better that is combinatorically so many more zeros. There was a conference that Amy and I were at, the Data Event Conference where I was talking to one of the big pharma CEOs and he threw out this factoid that there are 10,000 trillion organic compounds with a certain number of atoms and there's only 4,000 approved drugs in the whole planet.
Marty Chavez: So there's many zeros in between there. And I'm just going to say that that the machines are getting so much faster and the algorithms and the data sets are getting so much better. And you know, Amy, we're seeing this right now, the things that are coming out with generative AI every three months are twice as interesting as what was out the month, the time before. I think this is all coming together and we're going to have some real breakthroughs in making people better as all these data sets come together with all these algorithms and all this compute power here. What do you think, Amy?
Amy Abernethy: I totally agree.
David Stiepleman: Well, let me finish on this. It sounds like our regulator is in a good shape to be a real partner in advancing that. And you can kind of see that I think, again, as a non-expert through Covid, like you, all the things you were talking about, I'm not sure as a society we covered ourselves in glory through Covid, but the FDA did and the public private partnerships did, and we got a vaccine reasonably quickly and we're kind of back on our feet. And so not to be corny, thank you. Thank you for your service and thanks for doing this conversation, which is at least as important as that. So, it was awesome. I really appreciate it. Thank you. And Marty, thank you.
Marty Chavez: Thank you so much, Amy. Thank you, David.
David Stiepleman: That was Dr. Amy Abernethy. Marty Chavez, and I spoke with Amy on Wednesday, December 21st, 2022. Usually we boiled down the episode into a few key learnings, but I think the conversation you just heard is so full of knowledge and wisdom. I'll just call out one. Amy's career path is very varied, of course, but it's also been a relentless pushing from various angles on how to get breakthroughs from the lab into patients who need them while maintaining rigor and safety. And she's been following that mission wherever it leads, regardless of what her path was “supposed” to be. I loved her talking about how you end up taking learnings and muscle memory from one stop into unexpected new realms. We are all the beneficiaries of her focus and insight. Second, I thought her vision of the regulator industry relationship where there's collaboration across that line to make the neighborhood better for everyone is a great model.
David Stiepleman: It's a symbiotic relationship when you have people of good faith on both sides of the table. And finally, the possibility of using data to simulate real world conditions like Marty talked about in a financial firm. And through the great financial crisis and the parallels to using real world data to innovate in the life sciences in terms of new technologies and better delivery mechanisms. I figured we'd have something to talk about there, but it was even more fruitful than I would've hoped. And getting an expert's look into where we are in terms of computing power and the possibilities of data use made me both nervous and super excited for what's next. And I appreciated Amy's focus on both the ethical issues and the vision of how this can all come together for the betterment of the world. Thank you again to Dr. Amy Abernethy for joining the podcast and her collaboration with Sixth Street and of course, my partner Marty Chavez for joining the show. It's always a better time if Marty's with you. You've been listening to ‘It's Not Magic’, a Sixth Street podcast. You can read more about our guests on Sixth Street.com and subscribe wherever you get your podcast. If you enjoy today's podcast, please share it and follow @SixthStreetNews on Twitter from more on the show and our firm. Thanks to Sixth Street's production team, Patrick Clifford and Ritvi Shah, putting this together with sound engineering by Stephen Colon. Our theme song is, ‘It's Not Magic’, an Original Creation by Patrick Dyer Wolf. Once again, I'm David Stiepleman. Thanks for listening.
David Stiepleman: The views expressed in this podcast are not necessarily those of Sixth Street and Sixth Street is not providing any financial, economic, legal, accounting, or tax advice. Or recommendations in this podcast. Please see additional disclosures on our website for more details.